Escape AI Pilot Purgatory the BORING WAY
Two years after ChatGPT sparked the AI boom, many companies remain stuck in 'pilot purgatory,' chasing trends but failing to realize value.
The solution?
A disciplined approach that combines Lean principles with frameworks like BORING (explained in detail later in this article) helps organizations uncover high-impact opportunities for AI automation. By focusing on customer value and team empowerment, businesses can finally escape the endless cycle of pilot projects.
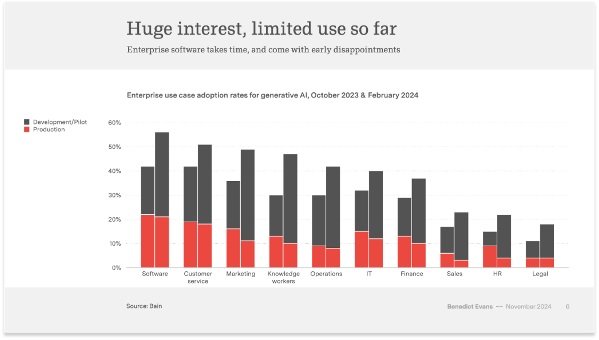
The Evolution of AI: From Oversimplification to Integration
Many businesses approach AI with an oversimplified view: input data, apply AI, and expect value to emerge seamlessly. This "black box" mindset misses critical steps in ensuring AI delivers tangible outcomes.
Traditional Machine Learning Processes
Effective AI requires rigorous data preparation, robust modeling, and careful operationalization. Unlike the oversimplified view, this process involves:
- Data Engineering: Data cleaning, transformation, and integration to ensure quality inputs.
- Modeling: Applying machine learning techniques to train, test, and validate models.
- Operationalization: Deploying models and continuously refining them based on real-world performance.
This process creates a foundation for actionable and reliable AI systems.
The GenAI-Enabled Process
Generative AI takes this evolution further, embedding itself into workflows and requiring guardrails, continuous monitoring, and human oversight. This framework prioritizes:
- Workflow Experimentation: Experimenting with AI, APIs, machine learning, and coding to refine processes.
- Guardrails: Establishing clear boundaries to ensure AI aligns with business goals and mitigates the risk around this new class of models.
- Human in the Loop: Empowering teams to monitor and refine AI outputs, ensuring continuous improvement.
Common Challenges
For decades, well before AI was part of every executive’s vernacular, businesses have rushed to adopt new technologies, hoping for transformational results. Yet many of these initiatives failed to deliver value, often stalling after costly pilot programs or proof-of-concept experiments.
AI adoption today faces similar challenges. Why do so many efforts fall short?
- Disconnected Processes: Many of the AI use cases we see are focused on isolated inefficiencies, such as automating a single task, without optimizing the entire value chain. For example, automating customer inquiries might reduce response times but create bottlenecks if the resolution process downstream isn’t integrated. This piecemeal approach limits AI’s impact and introduces new inefficiencies.
- Data Gaps: Poor-quality, incomplete, or siloed data undermines AI’s ability to deliver actionable insights. While 9 out of 10 business leaders believe they're ready for AI at scale, 70% of their technologists are spending hours each day fixing data issues. Without high-quality, connected datasets, AI is like a race car running on low-grade fuel.
- Neglecting Human Empowerment: In many organizations, AI is a technology searching for a problem. True transformation doesn’t come from technology alone. It happens when teams closest to the work are equipped to interpret AI outputs, refine systems, and drive continuous improvement. Without this empowerment, AI becomes a black box, disconnected from the people it’s meant to serve.
To avoid these common pitfalls, businesses must rethink their approach. Successful AI adoption requires the same discipline and holistic mindset that Lean principles demand, starting with a laser focus on understanding and delivering customer value. Instead of treating AI as a quick fix, organizations must view it as a tool to create seamless, integrated processes that amplify both human and machine capabilities.
“AI is Lean for knowledge work.” -Satya Nadella
Applying Lean Principles with AI
Lean's foundational principles provide a structured approach to scaling the potential of AI:
1. Start with Customer Value
Begin by understanding what customers need, not just at a superficial level, but deeply study those needs. Too much attention is spent on the flashy parts of AI’s potential. Getting in the hearts and minds of your customers and users, open up practical applications, like optimizing compliance workflows, simplify experiences for customers and drive measurable business value. After all, whether a process is manual or automated, it is still how value is delivered to customers. Tools like customer journey mapping, the Kano Model, and Lean value analysis help to build empathy for the customer and your team delivering that experience.
Building a holistic view of the customer/user’s needs.
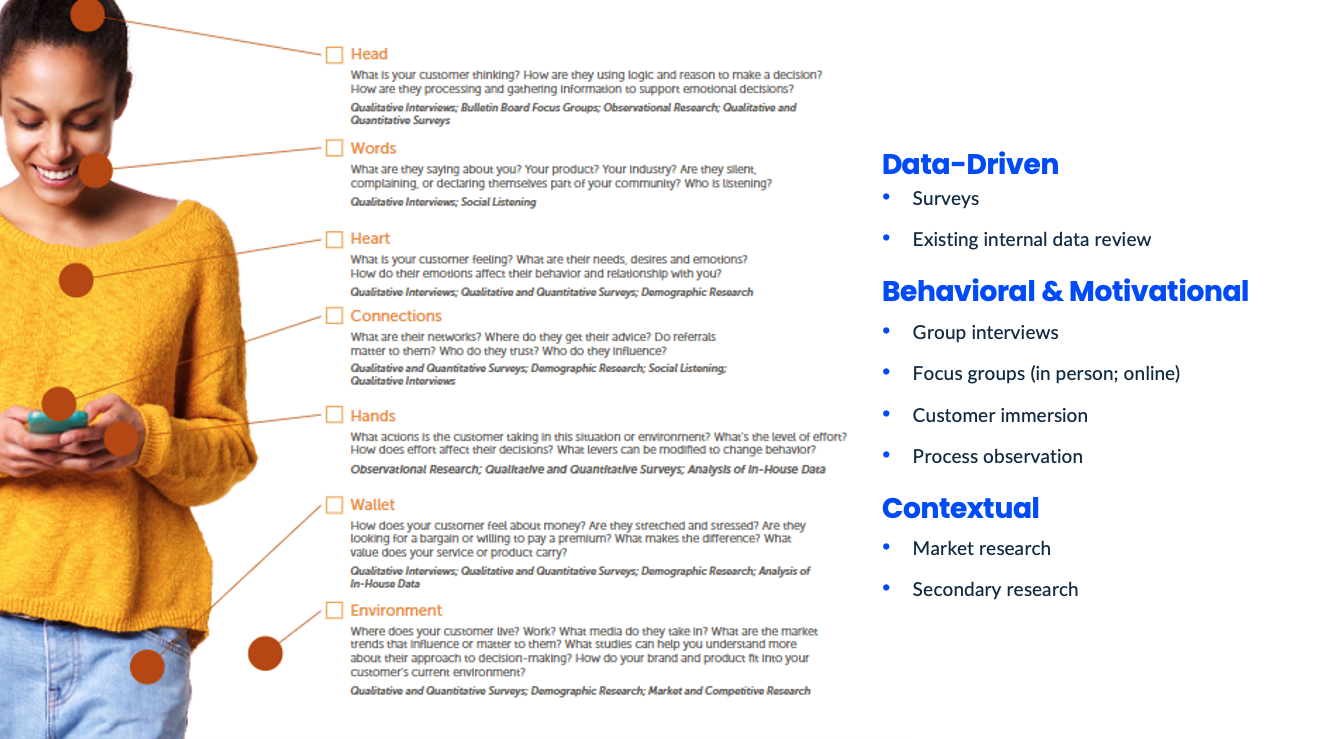
2. Connect Processes End-to-End
Customers don't care about your org structure or functional silos. It turns out that AI also thrives when workflows are integrated. Identify where processes break down, where data drops, and how systems can connect to deliver seamless outcomes. Seeing and understanding how your current processes connect to deliver customer value lays the groundwork for connecting disparate data sources and AI use cases, a prerequisite to realizing a vision where AI agents collaborate with other agents and their human teammates.
3. Optimize Flow with the Team
Knowledge workers are more stressed at work than ever before. They're struggling to keep up with communications from multiple channels, flipping between 5-6 systems to answer a customer inquiry, having to redo something because the data provided from one of the systems was inaccurate, and now there is the threat that AI will replace their jobs. Lean teaches us to engage the team in seeing, understanding, and incrementally removing waste from the system. AI can, then, be an amplifier by:
- Automating repetitive tasks like data entry.
- Providing real-time insights in the hands of the team, reducing delays and customer frustration.
Underwriters in the Excess & Surplus (E&S) insurance market often face delays of up to 5 days waiting on broker submissions buried in inconsistent emails and attachments. SingleStone recently partnered with a client to develop a tool using Microsoft Power Platform, Python, Docker, and Azure AI to extract, organize, and present submission data within minutes. This solution not only reduces turnaround time but also provides underwriting managers with full visibility into all submissions, empowering them to make faster, more informed decisions while fitting seamlessly into insurers’ existing Microsoft ecosystems.
4. Build Quality into Systems
A core tenet of Lean is incorporating quality into the process rather than relying on checks and QA steps to catch defects later. A major challenge in AI adoption today is data quality.
One client’s new hire onboarding process included 45% manual reviews and multiple rounds of rework, causing frustration for the new hire and the team working to onboard the hire. The client’s team was excited by the possibilities as we partnered to redesign the process with the supporting architecture, data foundation, and automation capabilities to eliminate all of this rework. While the efficiencies and scalability benefits are clear, the greatest value was in freeing the team to focus their creative energy on imagining new ways to bring their culture to life through the onboarding process instead of daily firefighting.
Similarly, AI must be designed with trust and transparency from the start. Invest in robust data governance and model risk management to ensure AI capabilities are accurate, unbiased, and reliable.
5. Empower the Team
AI amplifies human potential when teams that are closest to the work are equipped to adapt and refine systems. Engage the team in problem-solving for the customer and optimizing the process. Train teams to:
- Identify waste in the process and identify areas for improvement.
- Understand AI capabilities.
- Understand AI outputs.
- Collaborate with technology to drive innovation.
Our team recently partnered with a global financial services company’s team to assess and redesign its customer complaints process. Working side-by-side with the cross-functional process team provided rich opportunities to discover alternative approaches to optimizing for faster complaint resolution through automation and AI capabilities. The resulting process eliminated 50% of the manual effort causing customer and agent frustration, significantly reduced the operating expenses, and created long-term scalability as the client’s business executed its growth plans.

6. Commit to Continuous Improvement
Customer needs adapt and so must processes that support them. AI solutions should also evolve alongside business needs, especially with the rapid pace of change in the technology. Establish feedback loops to refine models and optimize performance over time.
Getting Started: Lean Meets AI
The first step in adopting AI with Lean principles is understanding the end-to-end customer journey. Here’s a roadmap for getting started:
1. Assemble a Cross-Functional Team
Bring together stakeholders who own different parts of the process to ensure alignment and collaboration. Remember, customers don’t care about your internal silos. They care about seamless experiences.
2. Map the Current Process
Create a detailed map of the customer journey and internal processes, identifying where data is generated, used, and stored. Highlight any silos or inefficiencies. This is also the time to understand what the customer values in the process. The Kano Model can help with prioritizing what the customer values and how the current process is meeting those needs.
3. Identify Key Drivers of Waste
Evaluate each step as value-adding to the customer, business-necessary, or non-value-adding. Be relentless about removing non-value add steps. Lean identifies eight forms of waste with the handy DOWNTIME acronym. These “waste” activities are necessary today because of the existing processes, technology, and data. While necessary, it’s these non-value add activities that create frustrating experiences for customers and the team mired in this waste trying to serve those customers. Imagine the human potential if our teams stopped wasting our time in these areas and could focus on driving customer value.

4. Establish Metrics and Baselines
Define clear KPIs for customer value, operational efficiency, and business outcomes. Establish baselines and set realistic targets for improvement. Ladder these metrics into your overall dashboards to create the linkages between expected AI improvements and customer and business outcomes.
5. Redesign for Flow and Value
Optimize workflows to align with customer needs and eliminate waste. This is where AI (and other tech) comes in. Identify where AI can eliminate non-value add activity and help to automate the customer and business value add activity. Not only does this optimize for customer value but it also removes the human toil and frustration in the daily lives of the team that's mired in serving the customer as best they can within today's suboptimal process.
For example, we worked with a client to remove greater than 50% of the manual effort from their customer servicing process through AI and automation, significantly reducing time to customer resolution and increasing the future scalability of the process.
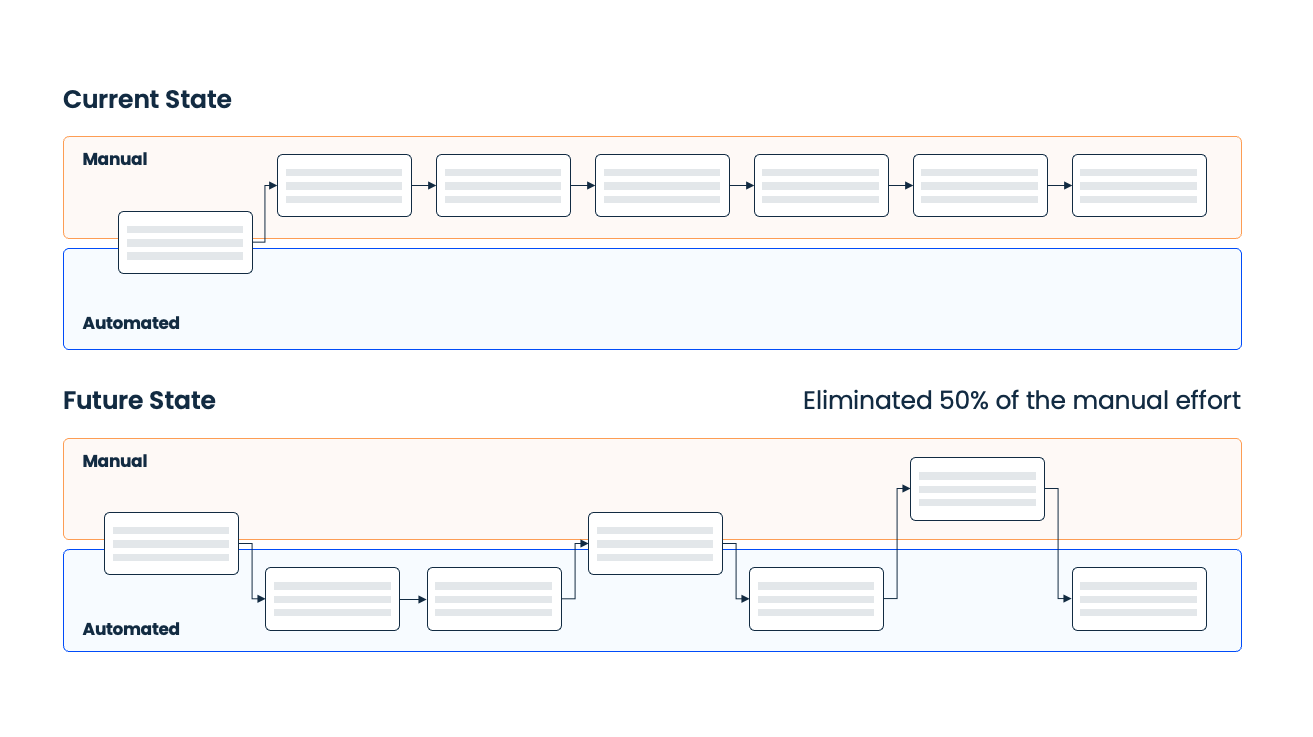
6. Prioritize Use Cases
Focus on high-impact opportunities where AI can deliver measurable customer and business value. Prioritize these based on feasibility and potential ROI. See the BORING framework below for a collaborative approach to prioritization.
7. Implement, Monitor, and Iterate
Deploy AI solutions incrementally, measure their impact, and refine both the technology and the process through continuous improvement cycles.
Identifying Opportunities for AI Automation: The BORING Framework
To prioritize processes for AI-driven automation, use the BORING Framework, evaluating each process based on:
- Business Valuable: Does this process directly impact revenue, customer satisfaction, or competitive advantage?
- Operationally Important: Is this process critical to the organization’s day-to-day functioning?
- Repetitive: Does this process involve repetitive tasks that are time-intensive and prone to errors?
- Integral: Is this process interconnected with other workflows or systems?
- Navigational: Does improving this situation, or loosening the bottleneck a little bit (goal is to be better, not precisely right) result in business impact?
- Growth Enabling: Can improving this process create measurable scalability or efficiency gains?
By scoring processes against these criteria, leaders can identify high-impact opportunities for AI automation that align with business objectives.
Want to take it a step further? Check out our Miro board on running a BORING Workshop to effectively identify and prioritize automation opportunities in your organization.
Empowering the Future of Knowledge Work
The true promise of AI lies not in just replacing tasks but in enhancing the way people solve problems and serve other people. By applying AI thoughtfully, with a focus on customer needs, seamless workflows, and team empowerment, businesses can reshape knowledge work and unlock meaningful progress.
Transformation begins with a clear and intentional step: identifying where technology can amplify impact while remaining grounded in the needs of the people it serves. For organizations stuck in pilot purgatory, caught in cycles of experimentation without meaningful outcomes, this disciplined, people-centered approach offers a way out. By embracing frameworks like BORING and applying Lean principles, businesses can break free from stagnation, set a clear course for scalable success, and ultimately redefine the standard for what’s possible in tomorrow’s knowledge work.
Where will your organization begin? Where will your organization begin? The time to start is now. Map your workflows, uncover inefficiencies, and take that first step—perhaps with a BORING Workshop—toward unlocking the transformative power of AI.
Written by Jimmy Chou CEO of SingleStone Consulting